How to Remove Seasonal Fluctuations for Better Financial Analysis
- Posted in Budgeting / Financial Analysis
- 12 mins read
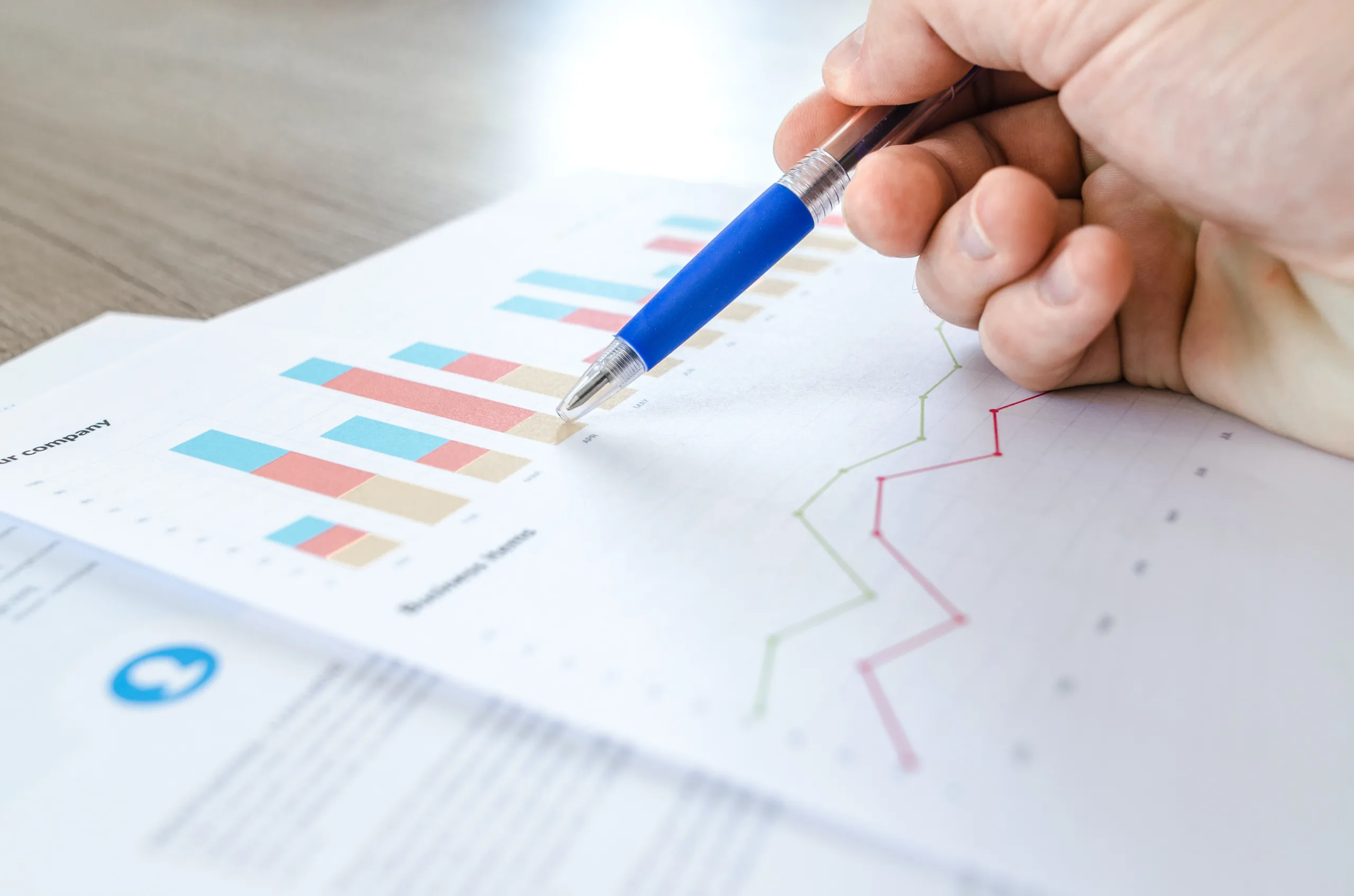
In business, accurate data interpretation is critical. Data guides everything from company strategies to investment decisions. However, data can sometimes be misleading if not viewed in the correct context. This is especially true when external factors, like seasons or holidays, influence the numbers.
Imagine a company that sells ice creams. Their sales figures will likely soar in the summer and plummet in the winter. If an analyst, unaware of this business’s seasonal nature, looks at the winter sales figures, they might think the company is in trouble. Conversely, if they only viewed the summer figures, they could overestimate the company’s overall success. This is a classic example of how seasonal fluctuations can paint an inaccurate picture of a business’s health.
Seasonality can also obscure trends. When the numbers move up and down regularly due to seasonal factors, it is harder to see the underlying trajectory of the big picture. With enough hindsight, you can see the larger pattern, but by then, it may be too late to respond to the changing environment.
Here’s where the concepts of deseasonalizing and reseasonalizing come into play. These are essential tools in the financial analyst’s toolkit. By understanding and accounting for seasonality, analysts can strip away external influences and see the real performance and potential of a business.
In the upcoming sections, we’ll delve into how to identify seasonality, the process of deseasonalizing, and situations where reseasonalizing data makes sense. By the end, you’ll have a clear grasp of why these techniques are vital for accurate financial analysis.
Understanding Seasonality
Seasonality refers to predictable and recurring changes in data that occur at specific periods. For example, retail businesses might see a sales spike every December due to holiday shopping. If analysts only looked at this data, they might mistakenly think the business is growing at an exceptional rate. To get a clear picture, it’s crucial to remove these seasonal effects.
Deseasonalizing Financial Data
Deseasonalizing, sometimes called “seasonal adjustment,” is the process of removing seasonal effects from data.
Steps to Deseasonalize Data:
- Identify the Seasonality: Before you can remove seasonality, you need to recognize it. This usually requires analyzing data across multiple periods (e.g., months, quarters, years).
- Calculate the Seasonal Factor: This is a measure of how much the data typically increases or decreases during a seasonal period. It’s found by averaging the seasonal effects over multiple periods.
- Adjust the Data: To remove the seasonal effect, you divide (for multiplicative models) or subtract (for additive models) the seasonal factor from the actual data.
Reseasonalizing Data
After analyzing deseasonalized data, there might be situations where it’s useful to reintroduce the seasonal effects. This process is called reseasonalizing.
Why Reseasonalize?
For future projections, knowing the raw, seasonal figures can be essential. For instance, a company planning its inventory might need to prepare for seasonal spikes in demand.
Steps to Reseasonalize Data:
- Retrieve the Seasonal Factor: This is the same factor you calculated and removed during deseasonalization.
- Apply the Factor to Deseasonalized Data: You multiply (for multiplicative models) or add (for additive models) the seasonal factor back to the deseasonalized figures to get the reseasonalized data.
Benefits of Deseasonalizing and Reseasonalizing
- Better Analysis: By removing seasonal fluctuations, analysts can get a clearer picture of a company’s true performance.
- Accurate Projections: Deseasonalized data helps identify underlying trends, which can be crucial for forecasting.
- Informed Decisions: Reseasonalized data ensures that businesses are prepared for seasonal changes in demand or supply.
Deseasonalizing and reseasonalizing financial data are essential techniques for any financial analyst. By understanding and adjusting for seasonality, businesses and investors can make more informed decisions and get a clearer picture of financial performance.
Why Does Seasonality Matter?
The influence of seasonality on financial data is so substantial that, if ignored, it can lead to misconceptions about a company’s performance. Here are some reasons why seasonality is important:
- Business Strategy Development: Companies must understand their seasonal trends to optimize inventory management, staffing levels, and marketing efforts. For example, a toy store might ramp up its inventory before the holiday season, expecting higher sales.
- Investor Expectations: Shareholders and potential investors monitor company performance closely. Seasonal highs can falsely inflate expectations, while seasonal lows might raise undue concerns. By understanding seasonality, investors can make more informed decisions about buying or selling stocks.
- Budgeting and Financial Planning: For budgeting purposes, it’s crucial for businesses to understand when they’ll likely see more revenue coming in and when expenses might rise. This knowledge helps in allocating resources efficiently throughout the year.
- Benchmarking and Comparisons: When businesses compare their performance to competitors or industry averages, accounting for seasonality ensures a more apples-to-apples comparison.
Identifying Seasonality: Not All Businesses Are the Same
While some seasonal patterns, like increased retail sales during the holiday season, are widely recognized, others might be more subtle or unique to specific industries. A ski resort, for instance, will have its peak season during the winter months, while a beach resort might see its best numbers in the summer.
It’s also worth noting that seasonality isn’t just limited to sales. Other aspects of financial data, like production costs or operational expenses, can also exhibit seasonal trends. For instance, a company might see higher energy costs during colder months due to heating needs.
Seasonality is a pervasive aspect of financial data, and recognizing its influence is the first step toward effective financial analysis. As we proceed, we’ll delve into techniques that help strip away these seasonal effects, allowing for a clearer, more accurate view of a company’s performance.
A Closer Look at Deseasonalizing
When we talk about “deseasonalizing” financial data, we’re essentially discussing the process of filtering out those predictable seasonal fluctuations. This refined data provides a clearer view of a company’s underlying performance, separate from any external, cyclical influences.
Why Deseasonalize?
Before diving into the steps, let’s understand why we do it:
- Consistency: Deseasonalized data allows for a more uniform comparison across different time frames. For example, comparing sales from a typically slow month, like February, to a bustling month, like December, can be misleading without adjusting for seasonality.
- Trend Spotting: Removing seasonal effects makes it easier to spot underlying trends, whether they’re upward, downward, or stable. This is particularly valuable for long-term planning and forecasting.
- Efficient Resource Allocation: By understanding the true performance of a business, companies can allocate resources more efficiently, ensuring they’re not reacting to seasonal anomalies.
Steps to Deseasonalize Data:
- Identify the Seasonality: This goes beyond just recognizing it. Utilize historical data, preferably spanning several years, to pinpoint exactly when and how these seasonal effects manifest.
- Calculate the Seasonal Factor: This is an average measure of how much a data point (like monthly sales) increases or decreases due to seasonal influences. For instance, if sales in December are consistently 20% higher than the average, then 20% is the seasonal factor for December.
- Adjust the Data: With the seasonal factor in hand, the next step is to strip it from the actual data. Depending on the data model you’re working with, you’ll either divide the actual data by the seasonal factor (for multiplicative models) or subtract it (for additive models). The result is your deseasonalized data.
It’s worth noting that specialized software and tools can assist in deseasonalizing data, especially when working with vast datasets. These tools can automate much of the process, but understanding the underlying principles remains crucial for accurate interpretation.
Calculating Seasonal Factors: A Step-by-Step Guide
Understanding seasonal factors is essential in the process of deseasonalizing financial data. In this section, we’ll break down the process of calculating seasonal factors in a manner that’s both detailed and accessible.
- Gather Historical Data:
Start by collecting historical data for the variable you want to analyze. For instance, if you’re studying monthly sales, gather sales figures for multiple years. The more data you have, the more accurate your seasonal factor calculation will be.
- Calculate the Average:
Find the average of the collected data over a consistent period. For monthly data, calculate the average monthly figure over the entire dataset.
- Determine the Seasonal Effect:
For each period in your dataset (e.g., each month), divide the actual data by the average calculated in the previous step. This will give you a ratio that represents the seasonal effect for that period.
For example:
- If the average monthly sales is 100 units and December sales are 120 units, the seasonal effect for December is 1.20 (120 ÷ 100).
- Calculate the Average Seasonal Effect:
To ensure precision, it’s essential to calculate the average seasonal effect for each period over multiple years. So, if you have data for five years, sum up the seasonal effects for each December and then divide by five to get the average December seasonal effect.
- Review and Refine:
Once you have your seasonal factors for each period, review them for accuracy. Outliers or inconsistencies may indicate errors or unique events that should be accounted for. It’s good practice to periodically revisit and update these factors as new data becomes available or market conditions change.
- Apply Seasonal Factors:
With the seasonal factors in hand, you can now use them to deseasonalize your data. To remove the seasonal effect from raw data, you would either divide (for multiplicative models) or subtract (for additive models) the seasonal factor from the actual data.
Calculating seasonal factors is a foundational step in the process of deseasonalizing financial data. By following these steps and maintaining a rigorous approach, financial analysts and businesses can unlock clearer, more accurate insights from their data, setting the stage for informed decision-making.
Reintroducing Seasonality
After stripping away the seasonal fluctuations to get a clearer view of the data, there may come a time when reintroducing these effects is beneficial. This process, known as “reseasonalizing,” ensures that the real-world implications of seasonality are not lost, especially when planning for the future.
The Rationale Behind Reseasonalizing
Why might someone want to bring back the very fluctuations they removed? Here’s why:
- Future Projections: Deseasonalized data provides a smooth trend, but when planning for the future, businesses need to be prepared for the natural peaks and valleys. For instance, if a company is setting inventory levels, they’ll need to account for the expected seasonal surges.
- Stakeholder Communication: Stakeholders, whether they’re investors, partners, or employees, might be more familiar with the seasonal data. Presenting them with reseasonalized figures can align communication and avoid confusion.
- Operational Decisions: On-the-ground decisions, from staffing shifts to marketing campaigns, often need to factor in the immediate, season-impacted future. Reseasonalized data provides that context.
Steps to Reseasonalize Data:
- Retrieve the Seasonal Factor: Remember the seasonal factor you calculated and removed during deseasonalization? You’ll need that again. It’s the key to re-introducing seasonality.
- Apply the Factor to Deseasonalized Data: With your seasonal factor at the ready, it’s time to weave the seasonality back into the data. If you’re working with a multiplicative model, you’ll multiply the deseasonalized data by the seasonal factor. If it’s an additive model, you’ll add it back.
Ensuring Accuracy in Reseasonalizing
Accuracy is paramount. When reseasonalizing data, it’s essential to ensure that the seasonal factors used are up-to-date and relevant. Seasonal trends can shift over time, so continuously revisiting and recalibrating is a good practice.
Reseasonalizing is not about going backward but about ensuring the data is versatile and applicable in various scenarios. With a firm grasp of both deseasonalizing and reseasonalizing techniques, financial analysts can navigate the complexities of financial data, providing insights that are both deep and broad.
How Deseasonalizing and Reseasonalizing Enhance Financial Analysis
Navigating the world of financial data requires precision and clarity. The techniques of deseasonalizing and reseasonalizing serve as tools to ensure that the data tells an accurate and actionable story. Let’s delve into the benefits of using these methods in financial analysis.
Clarity in Analysis
- True Performance Evaluation: With seasonal fluctuations removed, businesses and analysts can assess the genuine performance of a company, devoid of external influences. This can shed light on whether a business is truly growing, stagnating, or declining.
- Identifying Anomalies: Once the regular seasonal patterns are removed, any irregularities or unusual spikes in the data become more evident. Such anomalies might indicate specific issues or opportunities that could have been overlooked in the seasonal noise.
Enhanced Forecasting
- Predict Future Trends: With the clearer trend lines provided by deseasonalized data, it becomes easier to project future performance. Such forecasts can guide businesses in long-term strategies and investment decisions.
- Budget with Precision: By understanding the core performance trends, businesses can allocate budgets more effectively. This ensures that resources are directed where they’re most needed, without seasonal distortions misleading the allocations.
Informed Decision Making
- Strategic Planning: Knowing when to launch a product, start a marketing campaign, or make an investment becomes more strategic when the data is free from seasonal influences.
- Mitigate Risks: By reseasonalizing data, companies can prepare for seasonal downturns, ensuring they have strategies in place to weather slower periods.
Benchmarking with Confidence
- Competitive Analysis: When comparing performance with competitors, deseasonalized data offers a more level playing field. This ensures that companies are not misjudging their position in the market due to seasonal distortions.
- Industry Standards Alignment: Companies can better align with industry standards and best practices when they’re basing decisions on data that’s been refined to reflect true performance.
The techniques of deseasonalizing and reseasonalizing are more than just data manipulation. They’re about extracting the most meaningful insights from financial data. By refining data this way, businesses, investors, and analysts are better equipped to make decisions that drive success. As with any tool, the value lies in its application, and in the realm of financial analysis, these techniques are truly indispensable.
Share with: